One can argue that Europe is more inclined towards developing regulations than competitive AI models. As the EU formulates its AI Act to regulate AI within Europe, health AI projects are emerging that prioritize patient data protection. We spoke with the coordinator of the FEDERATED-PET project at the Lacassagne Hospital in Nice, Professor Olivier Humbert. 10 French hospitals including the oncology department in his hospital are building AI models using data from patients who have experienced lung cancer, all while ensuring that this data remains within the hospitals’ servers.
At the Sophia Summit, to which DirectIndustry was invited last month, Guillaume Avrin, National Coordinator for Artificial Intelligence at the French Ministry of Economy summarized the state of AI in Europe.
“Being the first would cost 10 times more than being second at developing this technology. But we want to be the first to develop disruptive technology, and for that, we are mobilizing our ecosystem. Investment in Europe for AI safety is billions of euros. We are ahead of the US on this. Europe can have leadership in AI safety. We have the expertise, but we lack visibility. We need to ensure that our achievements regarding AI trust are visible and identified as solutions.”
And there are several projects on safe AI in Europe, such as the French project FEDERATED-PET. This 1 million euro project, initiated one year ago and spanning a total of four years, focuses on developing the necessary methodology to extract information and data from PET imaging data, in applications related to lung cancer. This comprehensive AI for lung cancer detection will use AI models created within 10 French hospitals by the doctors themselves.
Professor Olivier Humbert is the Head of the Nuclear Medicine Department at the Centre Antoine Lacassagne in Nice. He is the coordinator of the project in collaboration with the French National Institute for Research in Digital Science and Technology (INRIA). He answered our questions.

For the FEDERATED-PET project to succeed, hospitals need to be actively involved. This is what Marco Lorenzi from the INRIA, with whom you collaborate, shared with us. How do you, in Nice, actively contribute to this?
Olivier Humbert: “Being actively involved means identifying patients eligible for the project and ensuring data quality. This involves a significant investment by doctors in the project because they are responsible for gathering the data. Often, in many AI projects, especially when they are not federated, they are centralized on hubs, and doctors are distant from these projects. The advantage of federated projects is that they occur within hospitals, where there is access to images and data.”

How does it happen concretely within the hospitals? What are the major steps for training the models?
Olivier Humbert: “Each hospital has formed a dedicated team with a designated lead physician who serves as the scientific head of the project for that hospital. We initiate the process by constructing a database for each hospital. In this particular project, we have 1300 patients distributed across 10 hospitals, averaging 130 patients per hospital.
The initial step involves each hospital identifying the 130 patients. There is an extensive review process led by medical professionals to ensure the selection of the right patients, those representing the target population for training the models. We work with data from patients who have already undergone treatment for lung cancer, examining the type of treatment they received to determine its effectiveness. Once the hospitals have identified this group of patients, they gather the clinical data, including weight, height, age, cancer type, and also the images such as scans taken before and after the treatment.
Visual inspections, such as a naked-eye examination of images and scans, are performed to verify that the correct and high-quality images are used. It’s crucial because, despite emphasizing the need for quantity in databases, having artifacts or low-quality data can introduce biases into the models. In my experiences with AI in predictive and diagnostic medicine, biases are a significant challenge. However, in a federated approach, the data within hospitals is handled by the medical staff who are familiar with it, allowing for a very clean quality control process. Then the medical team retrieves the information indicating whether the treatment was successful or not and deposits it onto a GPU server with the federated learning software developed by the INRIA.”
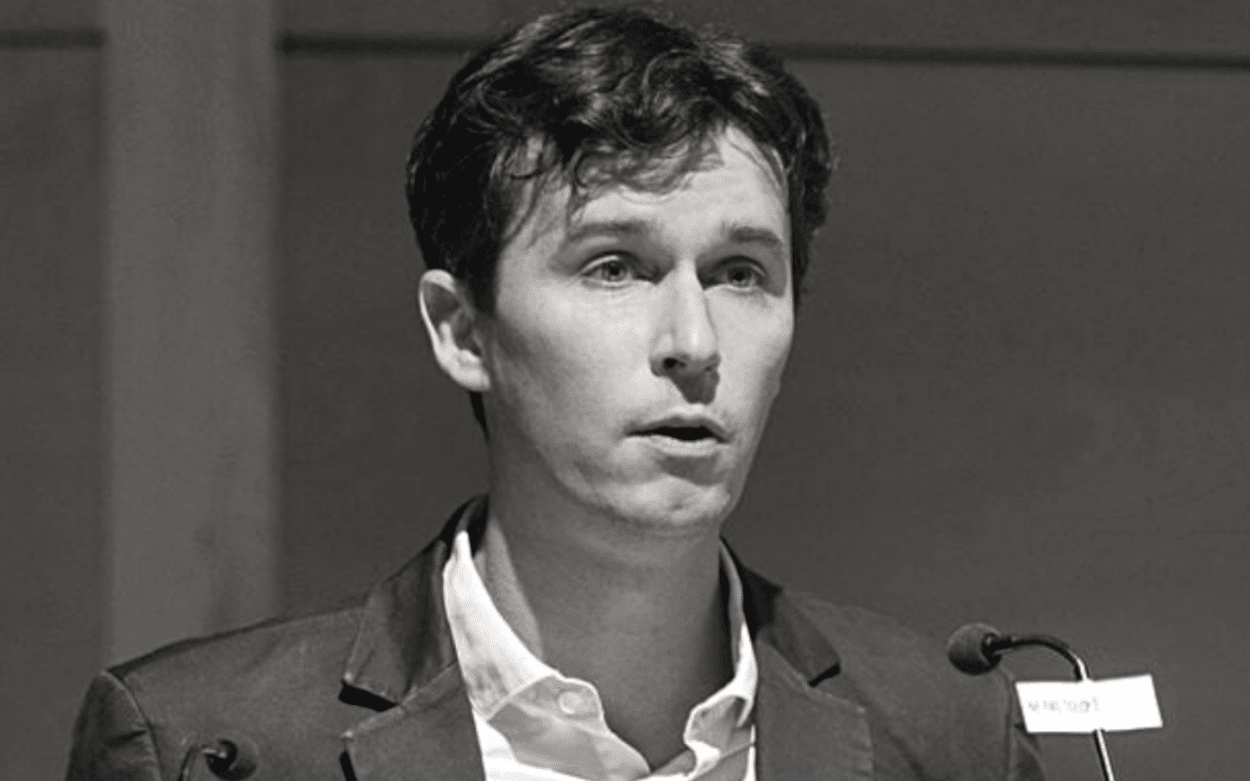
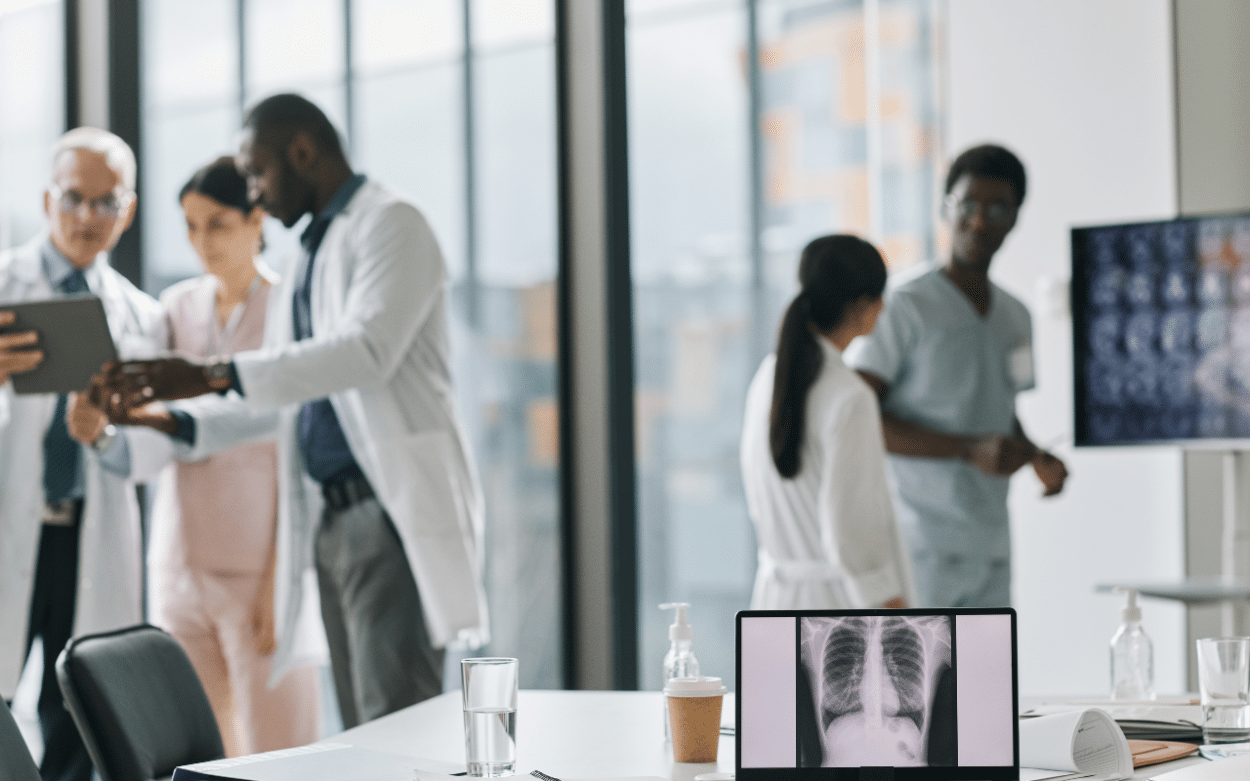

Do you rely on the existing IT team in the hospitals?
Olivier Humbert: “Of course, we leverage the hospital’s IT department, including the IT director (DSI) and the Data Protection Officer (DPO). Every hospital has an information systems department, but these IT professionals specialize in hospital networks. We don’t task them with AI responsibilities as it’s not their expertise. Instead, we ask them to install the necessary hardware for the project. This involves setting up a workstation with a computer and a dedicated, powerful GPU server capable of supporting AI training. Our IT teams install this powerful server within the hospital’s IT system, along with the software that facilitates communication with the federated network between hospitals and the INRIA. Even though we no longer exchange raw data, there’s still a centralized server located outside the hospital, at INRIA, enabling communication for each hospital to submit its AI models.”

What kind of equipment have you acquired for this project?
Olivier Humbert: “Each hospital is equipped with a GPU server. We also provide software for the hospitals because, for the learning process to work, the data from the hospitals must be structured in the same way. Currently, a PET scan from the Nice Hospital is not structured the same way as one from the Institut Curie. Therefore, we harmonize the software solutions for extracting and structuring images across hospitals to ensure uniform image processing.”

Are the doctors actively engaged in this project?
Olivier Humbert: “In this project, the participating doctors are volunteers who are enthusiastic and embrace innovation. What’s intriguing is that while there’s general wariness about AI, given the potential risks in hospitals, the federated concept has generated unprecedented enthusiasm for the project. The hospital and medical community love this project. Every time I presented it, people expressed a desire to be part of it.
A common criticism of AI projects in healthcare is that companies collect patient data, take it out of hospitals, and afterward, we lose visibility into the project’s progress, the algorithm’s training, how it functions, and how it has been evaluated. Waiting for a commercial solution might take five years, and that poses a real problem because it amounts to developing the medicine of tomorrow without close discussion with hospitals, doctors, and patient associations.
What doctors involved in the project appreciate with the federated approach is the deep involvement of hospitals. They actively participate, attending meetings every six months, assessing project progress, and verifying the quality of their data. There’s a strong emphasis on retaining control over the project. When the algorithm becomes transparent about encountered issues or its performance, we test it together with the doctors.
With federated learning, there’s first the security aspect of not extracting data from hospitals. Equally important is the idea of keeping medical teams at the heart of the project. Training occurs within the hospitals, and everyone appreciates this, as it ensures that the AI won’t escape our control. It enables us to use it for better patient care rather than for mere optimization.”
RELATED ARTICLE

What is the most challenging aspect for the doctors? They already have their daily responsibilities. Besides, the French hospital system is overstretched.
Olivier Humbert: “Indeed, doctors have immense workloads, so they must be willing and able to allocate time for this project, which is challenging. Among the ten projects, there are one or two hospitals that have withdrawn because it became apparent that the doctors were struggling, and the responsiveness wasn’t optimal. For the project to succeed, all 10 hospitals need 10 highly motivated doctors.
However, the most challenging aspect, in my opinion, lies with the hospital IT teams. IT professionals are few, and they are already overwhelmed with various issues, such as cybersecurity and urgent problems related to patient care. For instance, if a software issue arises and a critical system stops working, it means patient care is affected. Currently, there is no dedicated IT personnel specifically assigned to this research project. Doctors allocate some of their time, knowing this, but IT professionals are there to keep the hospital running smoothly, ensuring there are no cyber attacks. Fortunately, the project has a considerable number of engineers, including those from the INRIA, who liaise with hospital IT teams to assist.”

You’re a doctor and a medical researcher, but you seem to have a very good understanding of IT.
Olivier Humbert: “I had a keen interest in medical imaging, its analysis, and attempting to anticipate the success or failure of cancer treatments. So, about four or five years ago, I ventured into AI. I learned on the job by discussing with mathematicians and doing additional research. As a university professional, I already have a passion for innovation, research, and pushing boundaries. However, it’s complex, and it requires significant effort and investment. This is also why I implemented a training program, a university diploma for doctors, to educate them about AI. It involves 120 hours of courses delivered by mathematicians to help doctors grasp AI concepts. It takes place at my university in Nice and is open to all healthcare professionals, including dentists, pharmacists, and physiotherapists. So far, the doctors are quite satisfied.”

What do your colleagues think about AI in healthcare and what it could bring, especially in cancer detection?
Olivier Humbert: “Doctors want to stay informed about this topic. They sense a major turning point; it’s not just a passing trend. When I started with AI, people would say, “It’s just a trend.” No, it’s truly a technological revolution. Doctors have realized this. Being trained helps remove certain apprehensions. Many things about AI are somewhat mystified or feared irrationally. Understanding how AI works reduces biases and fear. It’s the first step. Additionally, I’ve observed that when doctors have less fear of AI, they tend to think it can solve everything, like magic. So, the second step is to make them understand that it’s not magic; there are real challenges. To avoid mistakes with patients, it’s crucial to know the limits of AI solutions and how to use them effectively. It’s about demystifying and highlighting the real limitations, emphasizing what to be cautious about when implementing an AI project to prevent errors in patient care.”