A new experiment at TU Delft explores the potential industrial applications of Large Language Models in co-developing cobots and guiding robotic design processes. DirectIndustry spoke with the researcher who led the study.
Since its public release in late 2022, ChatGPT has taken the world by storm. Open AI’s large language model has established itself as a revolutionary tool in many fields, showing a wide competency that writers, developers, and marketers, among others, are already employing to supercharge their work.
At the same time, ChatGPT and other Large Language Models (LLMs) showed clear limitations. They often spew out well-written and convincing misinformation or come up with hard-to-catch mistakes that make them unfit for high-risk applications in highly controlled and regulated fields.
While machine learning, deep learning, and computer vision already play an important role in the automation of the industrial sector, companies have been wary of adopting ChatGPT in wide-reaching tests, let alone in production. However, these new tools have already proven to be valuable companions for coders and developers and could help streamline a certain subset of activities, for example, helping in programming cobots and other industrial robots.
Designing Robots With ChatGPT
To explore how well LLMs would fare in such a scenario, researchers at the TU Delft in the Netherlands considered the case study of a human that leverages ChatGPT-3 to “help the world with robotics”. As described in an article published in Nature Machine Intelligence, they approached the task in two steps: first, in a high-level phase, the computer and human collaborate to conceptualize the project, discussing ideas and outlining the specifications for the robot design; in a second phase, the LLM is tasked with helping through the physical steps of programming the robot to achieve the desired result.
This way, ChatGPT helped researchers focus on the supply chain as a field where innovation could “help humanity” best. Thanks to the inputs from the LLMs, researchers picked tomato harvesting as the most economically valuable operation to automate.
Assistant Professor Cosimo Della Santina, the researcher who conducted the study along with Ph.D. student Francesco Stella, says:
“Here at TU Delft, we have been following the development of large language models for a while. When ChatGPT became available, we thought it was a great opportunity for a more solid analysis of their potential in the field of automation and if it could be used effectively for programming robots.
We expected the system to help in the middle of the pipeline, automating the robot’s design. Instead, we saw that the system could support us from the beginning, even in the conceptual phase. It only stopped when things needed to be drawings and get physical, so we took the design back in our hands from there.”
ChatGPT contributed meaningful inputs about some key aspects of the robot’s development. For example, it suggested using a silicon gripper for handling tomatoes to “reduce the risk of crushing the crops“, and to pick a Dynamixel motor as the best actuator for a tomato-harvesting gripper. According to Della Santina, the study wanted to understand how ChatGPT could help someone who doesn’t have previous knowledge of the field of automation:
“What we tried to do in this experiment is to pretend we knew absolutely nothing, or that at least we knew very little, much less than what we know, about engineering. We wanted to understand how far we could push the experiment, and we just made choices along the way, but always based on the options that ChatGPT gave us and the context it provided to help us choose. This was more interesting to us because it put ChatGPT in the position of directing the outcome, rather than having an expert user manage the LLM as it would an assistant.”
The experiment’s result was simultaneously surprising and proof that the field experience of humans is still needed. Interestingly, compared to other domains, ChatGPT didn’t make any glaring mistake that would have compromised the programming or creation of the robot. The researcher stopped only when they needed to move past the system’s current abilities to proceed to the actual fabrication of the robot.
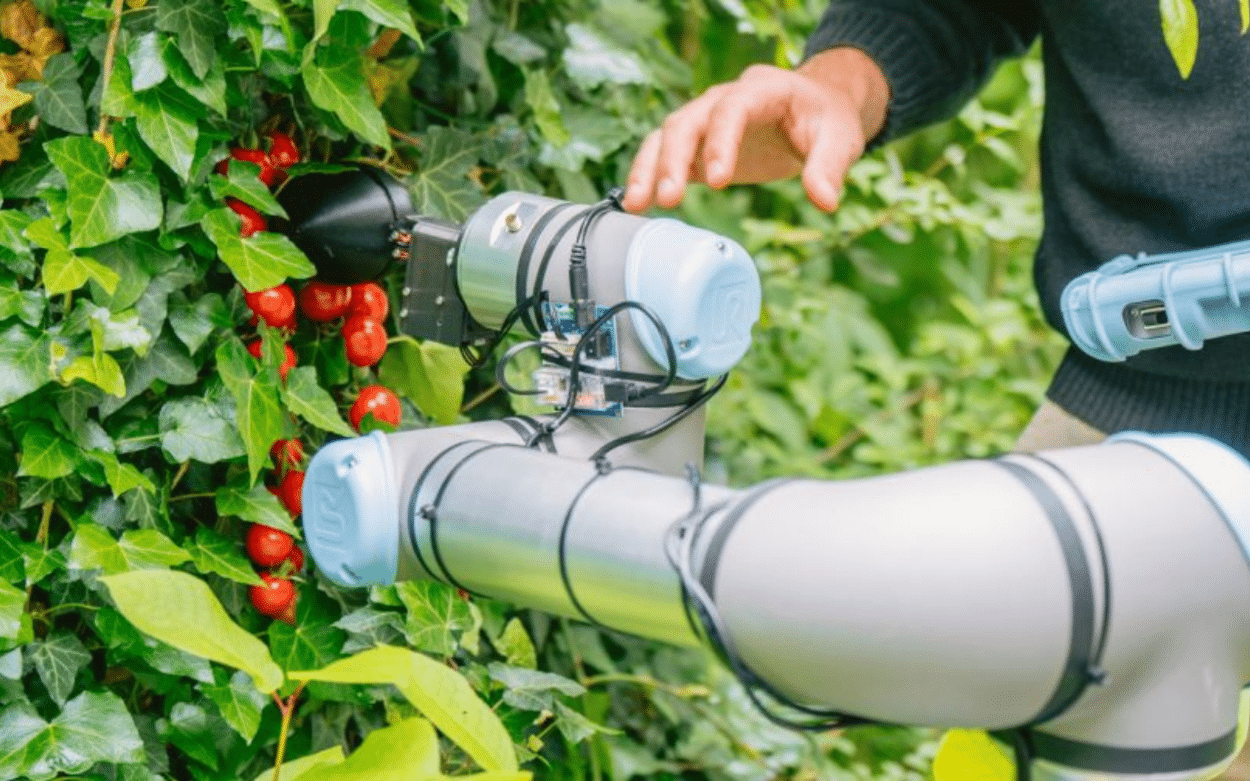
Replacing Engineers?
Despite the interesting outcome, Della Santina believes it’s still too early for systems like ChatGPT to substitute engineers. Still, it certainly gives advantages to smaller companies, which, until now, couldn’t afford the workforce needed to develop a solution like this tomato-picking robot.
“I don’t think we’re at any point where these systems could operate in the industry alone or unsupervised. A farming company would still be unable to employ ChatGPT instead of an engineer to automate its operations. What could soon happen, though, is that something that used to require two people with a certain level of knowledge now could need one with less expertise than two high-level engineers would have had before”.
While the integration of LLMs in the automation sector and the industry, in general, might still take time to happen (also for reasons related to security and privacy), many other technologies broadly bundled under the umbrella term of Artificial Intelligence have already been changing the game for quite a while. According to Della Santina, while LLMs have a yet unclear potential to disrupt the field of automation, other more practical applications of Deep Learning and Computer Vision have already found their way into industrial workflows and processes.
“One of the most interesting applications in robotics, for example, is learning by demonstration, which will have a high-impact direct application. Just think of kinaesthetic teaching systems, which allow a cobot’s task to be programmed through a learning-by-demonstration mode, in which the engineer or a technician takes the robotic arm and manually moves to ‘teach’ it what to do. With 20 or 30 task examples, the robot is then able to generalize and proceed to perform the task autonomously. That was the forefront of research ten years ago, but now these solutions have started to be certified and codified, and they’re reaching the market with prices that would justify the investment in automation even for smaller companies.”
But what would this mean for high-skilled workers? Will engineers and factory workers be displaced even more as soon as more AI-based solutions hit the market? Della Santina doesn’t shy away from this question, and despite his research being purely technological, he admits he’s also been thinking about these consequences.
“The way I see it, thanks to these new solutions, we could either keep the same number of workers and double the output or maintain the same output and keep half of the people. Technology gives us both options, and it’s up to us to want a choice that serves us all best”.